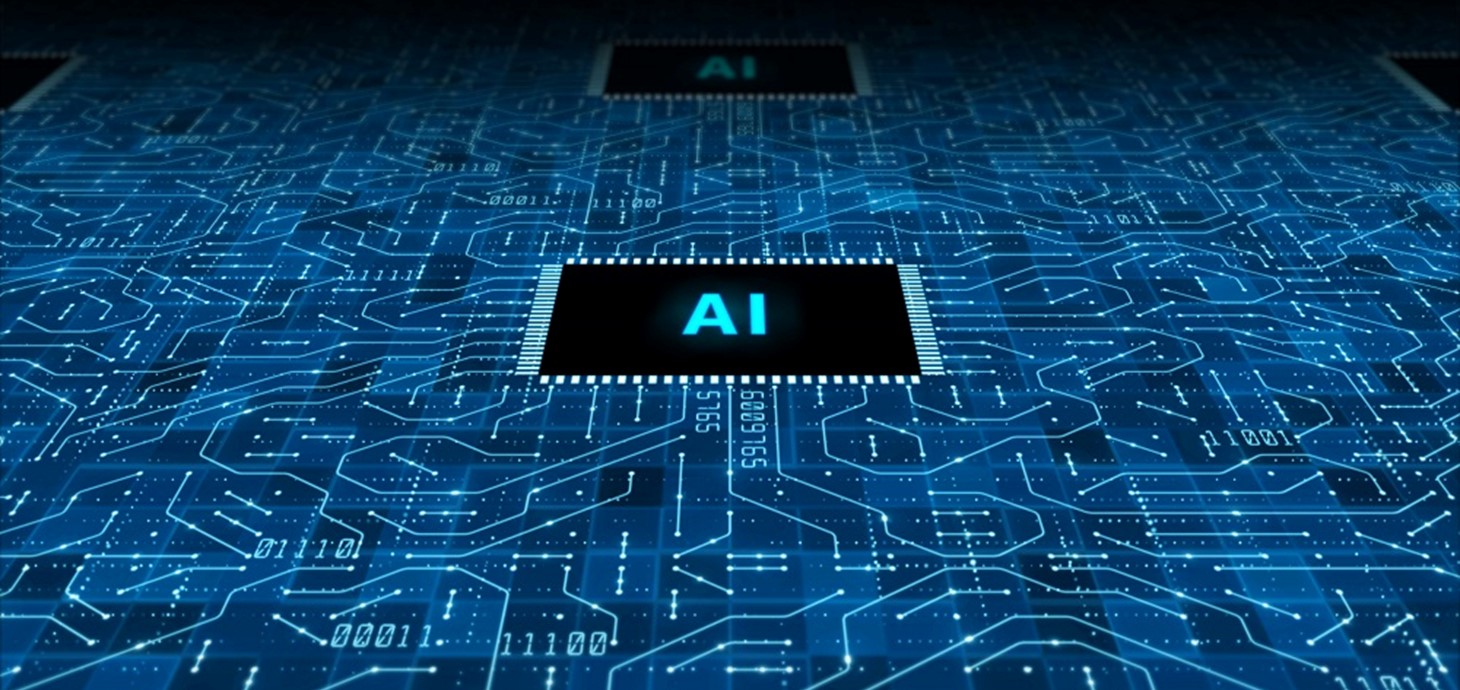
A research team at Swansea University have developed a ‘digital twin’ to help prioritise patients for urgent intensive care and ventilator support.
This new innovative system would mean patients are potentially seen more quickly and receive the most effective treatment based on data from previous pneumonia sufferers.
The team’s three-tiered system uses deep learning methods to build patient-specific digital twins to identify and prioritise critical cases amongst severe pneumonia patients.
A digital twin is a virtual representation (or computer programme) of a real-world physical system or product – it is updated from real-time data, and uses simulation, machine learning and reasoning to help decision-making.
Author and Associate Dean for Research, Innovation & Impact in the Faculty of Science & Engineering, Professor Perumal Nithiarasu comments:
“A human digital-twin is a digital replica of a human system or sub-system. This replica is a personalised digital representation, in terms of structure or functioning or both, of an individual or patient’s system.
It can provide real-time feedback on how a patient’s health is likely to vary based on their current known condition using periodic input data from the patient’s vitals (such as heart rate, respiration rate).
The severity scores calculated by these digital replicas (models), personalised to every patient using the individual’s vitals and other readings, can form the basis for prioritising potential pneumonia patients for Intensive Therapy Units (ITUs) and mechanical ventilation.
The three-tiered strategy proposed is used to generate severity indices to:
(1) identify urgent cases,
(2) assign critical care and mechanical ventilation, and
(3) discontinue mechanical ventilation and critical care at the optimal time.
The severity indices calculated in the present study are the probability of death and the probability of requiring mechanical ventilation. These enable the generation of patient prioritisation lists and facilitates the smooth flow of patients in and out of ITUs.
The proposed digital-twin is built on pre-trained deep learning models using data from more than 1895 pneumonia patients. Overall, results indicate that the prediction for ITU and mechanical ventilation prioritisation is excellent.
The data used to train the models is for non-COVID-19 patients with pneumonia. However this model may be employed in its current form to COVID-19 patients, but transfer learning with COVID-19 patient data will improve the predictions.”
Co-Author and IMPACT Fellow, Dr Neeraj Kavan Chakshu comments:
“The COVID-19 pandemic has put an unprecedented stress on an already strained healthcare infrastructure. This situation has forced healthcare providers to prioritise patients in critical need to access ITUs and mechanical ventilation.
Previous works on severity scoring use machine learning algorithms that were trained on large ITU datasets to calculate severity scores, covering a wide range of diseases and medical conditions. Such systems, though valuable during normal times, may not be sufficiently specific to address the pandemic.
In the case of COVID-19 (and in other similar forms of influenza), more precise and dynamically evolving system may be necessary to address the sudden increase in severity and the need for mechanical ventilation.
With potentially more mutations of the virus being identified over time, an evolving knowledge of the disease severity of each virus variant has become extremely important. A robust and dynamically adaptable model that takes into account the progression of severity over the course of care, which may be different for different variants of the disease, is therefore urgently needed.”
The paper, "An AI based digital-twin for prioritising pneumonia patient treatment", is published in the Journal of Engineering in Medicine.
The research was partially supported by Sêr Cymru III - Tackling COVID-19 programme, Welsh Government. The IMPACT operation is part-funded by the European Regional Development Fund through the Welsh Government and Swansea University.
Health innovation - Swansea University research